What is predictive analytics? An enterprise guide
Predictive analytics is a form of advanced analytics that uses current and historical data to forecast activity, behavior and trends. It involves applying statistical analysis techniques, data queries and machine learning algorithms to data sets to create predictive models that place a numerical value -- or score -- on the likelihood of a particular action or event happening.
Predictive analytics is a key discipline in the field of data analytics, an umbrella term for the use of quantitative methods and expert knowledge to derive meaning from data and answer fundamental questions about a business, the weather, healthcare, scientific research and other areas of inquiry. In the context of businesses, the main focus here, that process is often referred to as business analytics.
There are three major types of business analytics. The most common type is descriptive analytics, which gives an account of what has happened in a business. Predictive analytics, the subject of this guide, helps businesses predict what will likely happen. It looks for patterns in data and projects them forward to help businesses mitigate risks and capitalize on opportunities. The third category, prescriptive analytics, prescribes or automatically takes a next best course of action based on intelligence generated by the other two kinds of analytics. Two additional modes of analytics sometimes figure into the business analytics continuum: diagnostic analytics, which explores why something happened, and real-time analytics, which analyzes data as it's generated, collected or updated.
This guide to predictive analytics further explains what it is, why it's important and the business benefits it provides. You'll also find information on the tools and techniques used in predictive analytics, examples of its use in various industries, a five-step predictive analytics process to follow and more. Throughout the guide, there are hyperlinks to related articles that cover the topics in more depth.
One more bit of context before we dive in. Business intelligence systems -- which emerged in the early 1990s and by the 2000s were widely adopted by companies -- also help businesses make better decisions by collecting, storing, analyzing and reporting on past data. As BI platforms have evolved to accommodate big data and emerging technologies such as cloud computing, IoT and artificial intelligence, some people now consider business analytics a subset of business intelligence; others use the two terms interchangeably. Meanwhile, as machine learning has become fundamental to predictive analytics, many predictive analytics projects are simply referred to as machine learning or data science applications. The subtle differences and overlaps between these terms are important for experts to sort out, but in practice not so much. As business analytics authority Dursun Delen observed in his recently updated textbook on predictive analytics, "no matter the terminology used, the goal is the same: creating actionable insight from large and feature-rich data."
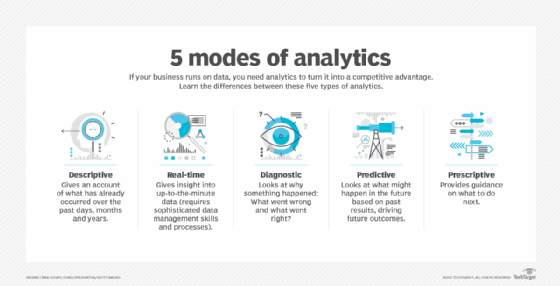
Why is predictive analytics important?
The need for predictive analytics is arguably more critical than it's ever been. "The traditional notion of learning from mistakes no longer applies; the reality nowadays is more like 'One strike and you are out,'" wrote Delen, a professor of management science and information systems at Oklahoma State University, in his introduction to Predictive Analytics, Second Edition. "The organizations that use business analytics not only can survive but often thrive in this type of condition."
Data is the lifeblood of business analytics and, increasingly, the fuel of business. Companies, big and small, run on data generated and collected from their operations and external sources. For example, companies collect data on every step of the buyer's journey, tracking when, what, how much and how frequently customers purchase. They also track customer defections, complaints, late payments, credit defaults and fraud.
But the massive amount of data businesses accumulate on their customers, business operations, suppliers, employee performance and so on is not useful unless it's acted on. "Data has become so ubiquitous in business operations that merely having access to more or better data is not in itself a key difference," noted analytics expert Donald Farmer, principal at consultancy TreeHive Strategy, in his in-depth article on the difference between descriptive, predictive and prescriptive analytics. "What changes business outcomes today is how we understand and act on our data. That understanding requires analytics."
Predictive analytics gives businesses a leg up by looking for meaningful patterns in this cumulative data, then building models that forecast what will likely happen in the future. For example, based on a customer's past behavior and the behavior of other customers with similar attributes, how likely is it that the customer will respond to a certain type of marketing offer, default on a payment or bolt?
Savvy sales and marketing departments have long taken advantage of predictive modeling, but the use of predictive analytics can now be found across business functions and industries. It is used tactically by organizations to improve key performance metrics by reducing risk, optimizing operations and increasing efficiency, and to set strategies that ultimately confer a competitive advantage.
However, advanced techniques like predictive analytics can be challenging, as outlined below.
How does predictive analytics work?
Predictive analytics software applications use variables that can be measured and analyzed to predict the likely behavior of individuals, machinery or other entities.
Multiple variables are combined into a predictive model capable of assessing future probabilities with an acceptable level of reliability. The software relies heavily on advanced algorithms and methodologies, such as logistic regression models, time series analysis and decision trees (see the section below on "Predictive analytics techniques").
Developing these forecasts is not necessarily easy, quick or straightforward. As Elif Tutuk, vice president of innovation and design at BI and data management software provider Qlik, told technology reporter George Lawton, the collection of data alone can take months or even years. Moreover, if the data is inaccurate or outdated, or the wrong tools are used, predictive outcomes will be negatively impacted.
The predictive analytics process varies by industry, domain and organizational maturity. A simple example of deploying predictive analytics involves buying a product -- for example, a fraud engine or spam filter -- that comes with predictive analytics capabilities and a mechanism for giving timely feedback to the people in charge of the service. On the other end of the spectrum are organizations that build robust frameworks for developing, releasing, deploying and iterating predictive models customized to their business.
How to develop a predictive analytics process
A detailed description of the key steps in deploying predictive analytics and the people skills required for them can be found in Lawton's article, "5-step predictive analytics process cycle." Here is a summary of each step:
- Define the requirements. Understand the business problem you're trying to solve. Is it managing inventory? Reducing fraud? Predicting sales? Generating questions about the problem and listing them in order of importance is a good start. Collaborating with a statistician at this stage can help form metrics for measuring success. A business user or subject matter expert generally takes charge of this first step.
- Explore the data. Here, you'll want to loop in a statistician or data analyst or both. The job is to identify the data that informs the problem you're trying to solve and the goal. Consider the relevancy, suitability, quality and cleanliness of the data.
- Develop the model. A data scientist can help figure out which predictive models are best suited to solving the problem. It's important to experiment with different features, algorithms and processes in order to strike a balance between performance, accuracy and other requirements, such as explainability.
- Deploy the model. Once the model is approved by the data scientist, a data engineer determines how best to retrieve, clean and transform the required raw data to deploy the model at scale and, above all, in a way that makes a meaningful difference -- e.g., integrating a new scoring algorithm into the sales team's workflow.
- Validate the results. Performance of the model can change over time due to shifts in customer preferences or the business climate, or unforeseen events such as a pandemic. Thresholds for updating models vary, requiring the joint expertise of a business user and a data scientist in this step.
The use and effectiveness of predictive analytics has grown alongside the emergence of big data systems. As enterprises have amassed larger and broader pools of data in Hadoop clusters, cloud data lakes and other big data platforms, they have created more data mining opportunities to gain predictive insights. Heightened development and commercialization of machine learning tools by IT vendors have also helped expand predictive analytics capabilities.
Also, just as BI tools evolved to become more user-friendly and therefore more widespread, the same trend is happening in advanced analytics. This topic is explored below in the sections on "Predictive analytics tools" and "The future of predictive analytics."
Still, deploying predictive analytics can be arduous, time-consuming and complicated -- and the benefits of this labor are by no means guaranteed.
These four points will help ensure success as you develop a predictive analytics strategy, advised TreeHive's Donald Farmer in his article, "Benefits of predictive analytics for businesses":
- Good predictions rely on good data. Incomplete or inaccurate data will not result in good projections.
- Good future outcomes depend upon choosing the best predictive modeling techniques when looking for patterns in data sets. Data scientists are trained in this, and new automated machine learning systems can run models to find the best approaches.
- Ambiguity is inevitable in predictions -- case in point: weather forecasts. Learn to work with imperfect results.
- Your predictions should be actionable insights. You should be able to do something useful with the prediction and test its accuracy in the future.
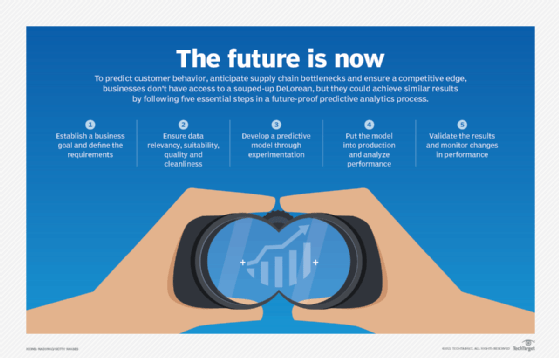
What is predictive analytics used for?
Weather forecasting is one of the best-known uses of predictive modeling. Predictive analytics is also used to forecast elections, predict the spread of diseases and model the effects of climate change.
In business, predictive modeling helps companies optimize operations, improve customer satisfaction, manage budgets, identify new markets, anticipate the impact of external events, develop new products and set business, marketing and pricing strategies. For example, an insurance company is likely to take into account potential driving safety variables -- such as age, gender, location, type of vehicle and driving record -- when pricing and approving auto insurance policies.
Business applications for predictive analytics include targeting online advertisements, analyzing customer behavior to determine buying patterns, flagging potentially fraudulent financial transactions, identifying patients at risk of developing particular medical conditions and detecting impending parts failures in industrial equipment before they occur. Wall Street uses predictive modeling to pick stocks and other investments.
As observed, the marketing industry has been a notable adopter of predictive analytics, along with large search engine and online services providers. Other industries that are big users of predictive analytics include healthcare and manufacturing. Specific examples of how companies use predictive analytics are detailed later in this guide.
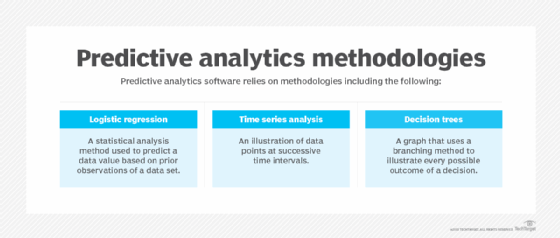
Predictive analytics techniques
Predictive analytics requires a high level of expertise with statistical methods and the ability to build predictive analytics models. As noted in the section on the five-step process for predictive analytics, it's typically the domain of data scientists, statisticians and other skilled data analysts. They're supported by data engineers, who help to gather relevant data and prepare it for analysis, and by BI developers and business analysts, who help with data visualization, dashboards and reports.
Data scientists use predictive models to look for correlations between different data elements in website clickstream data, patient health records and other types of data sets. Once the data collection has occurred, a statistical model is formulated, trained and modified as needed to produce accurate results. The model is then run against the selected data to generate predictions. Full data sets are analyzed in some applications, but in others, analytics teams use data sampling to streamline the process. The predictive modeling is validated or revised on an ongoing basis as additional data becomes available.
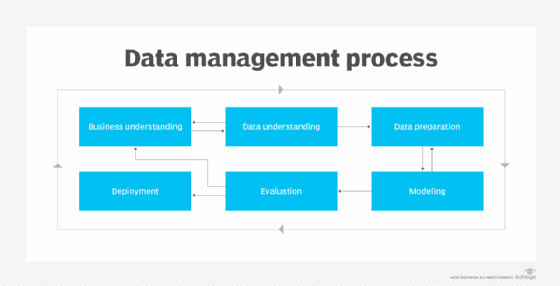
The predictive analytics process isn't always linear, and correlations often present themselves where data scientists aren't looking. For that reason, some enterprises are filling data scientist positions by hiring people who have academic backgrounds in physics and other hard science disciplines. In keeping with the scientific method, these workers are comfortable going where the data leads them. Even if companies follow the more conventional path of hiring data scientists trained in math, statistics and computer science, having an open mind about data exploration is a key attribute for effective predictive analytics.
Once predictive modeling produces actionable results, the analytics team can share them with business executives, usually with the aid of dashboards and reports that present the information and highlight future business opportunities based on the findings. Functional models can also be built into operational applications and data products to provide real-time analytics capabilities, such as a recommendation engine on an online retail website that points customers to particular products based on their browsing activity and purchase choices.
Beyond predictive modeling, other techniques used by data scientists and experts engaging in predictive analytics include the following:
- data mining to sort through large data sets for patterns and relationships that can help solve business problems through data analysis;
- text analytics to mine text-based content, such as Microsoft Word documents, email and social media posts;
- machine learning, including the use of classification, clustering and regression algorithms that help identify data patterns and relationships; and
- more advanced deep learning based on neural networks, which emulate the human brain and can further automate predictive analytics efforts.
What are examples of predictive analytics in business?
Examples of predictive analytics applications span business functions and industries. Moreover, as the technology becomes more accurate, easier to use and less expensive, the uses and benefits of predictive analytics will increase, reported technology journalist Maria Korolov in her updated look at the top predictive analytic use cases in business.
Here is a sampling of how businesses are applying predictive analytics.
Marketing. The use of predictive analytics in marketing has transformed how companies sell to customers. Technology writer Mary K. Pratt reported in her article on how to drive success in marketing with predictive analytics that the variety of use cases include next best action, lead qualification, proactive churn management, demand forecasting and "data-driven creatives" -- the use of predictive analytics to help decide what media style and form of messaging will resonate best with certain customers.
Supply chain management. The COVID-19 pandemic highlighted an increased need for better statistical models and forecasting in supply chain management. The pandemic forced companies to throw "historical data out the window," research analyst Alexander Wurm told Korolov, and to update their processes with real-time data and third-party information. For example, IoT-generated real-time data alerts companies to goods that have gone bad or been otherwise damaged, increasing the usefulness of predictive analytics in rapidly changing environments.
Fraud detection. The latest global crime survey from PricewaterhouseCoopers found that fraud rates are at record highs, costing companies worldwide a staggering $42 billion over the past two years. Many companies have small teams of investigators, making predictive technology essential to getting a handle on fraud. Predictive analytics is being used to scan through hundreds of thousands of insurance claims and refer just the ones that are most likely to be fraudulent to investigative teams. It's also being used by retailers to authenticate customers as they log in and monitor them for suspicious behavior as it happens.
Healthcare. As Pratt reported in her compendium of 12 ways predictive analytics provides value in healthcare, its use is both challenging and expected to increase in this field. Predicting the likelihood of patients developing certain medical conditions and forecasting the progression of diseases in patients are big uses, involving data stores from electronic health records, federal repositories, biometric data, claims data and more. Health administration also benefits from predictive analytics, which is used to identify patients at high risk for hospital readmission, optimize resource allocations and manage supply chains, among other applications.
Predictive maintenance and monitoring. IoT data is being used in predictive modeling to forecast equipment breakdowns. Manufacturers attach sensors to machinery on the factory floor and to mechatronic products, such as automobiles; the sensor data is then used to forecast when maintenance and repair work should be done in order to prevent problems. Predictive analytics is also used for monitoring oil and gas pipelines, drilling rigs, windmill farms and various other industrial IoT installations. Localized weather forecasts for farmers based partly on data collected from sensor-equipped weather data stations installed in farm fields is another IoT-driven predictive modeling application.
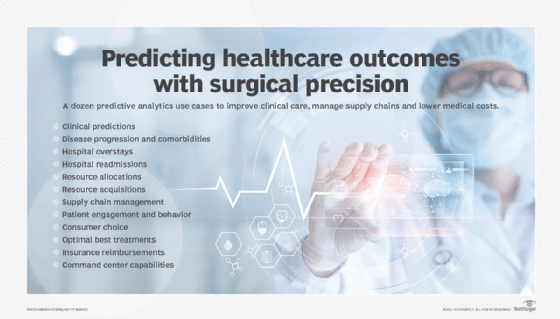
Predictive analytics tools
A wide range of tools is used in predictive modeling and analytics. AWS, Google, IBM, Microsoft, SAP, SAS Institute and many other software vendors offer predictive analytics tools and related technologies supporting machine learning and deep learning applications.
In addition, open source software plays a big role in the predictive analytics market. The open source R analytics language is commonly used in predictive analytics applications, as are the Python and Scala programming languages. Several open source predictive analytics and machine learning platforms are also available, including a library of algorithms built into the Spark processing engine.
Analytics teams can use the base open source editions of R and other analytics languages or pay for the commercial versions offered by vendors such as Microsoft. The commercial tools can be expensive, but they come with technical support from the vendor; users of pure open source releases, conversely, must troubleshoot on their own or seek help through open source community support sites.
Click on this link to read technology writer Lawton's report on how predictive analytics software is evolving and six top predictive analytics tools for 2022.
Predictive analytics market growing in size, importance
Projected to hit $10.5 billion this year, the market for predictive analytics is expected to nearly triple in size to $28 billion by 2026, according to Markets and Markets.
While staffing and budget issues related to the COVID-19 pandemic have put a hold on some companies' investment plans in analytics technology, for other companies, analytics has become even more critically important, helping enterprises navigate fast-changing customer behaviors and supply chain disruptions, according to the report.
The importance of predictive analytics and other types of analytics in the enterprise was evident in a 2021 survey of nearly 2,000 knowledge workers conducted by Hanover Research for LogiAnalytics: 90% of respondents said that analytics are "very or extremely valuable" when making business decisions, and 87% said their organizations used analytics "often or very often" to make business decisions.
What is the future of predictive analytics?
Traditionally the domain of data scientists and other quantitative experts, predictive analytics has been an art and science practiced by the highly trained few for -- when it works -- the benefit of the many.
But the field of advanced analytics is changing. As noted in Lawton's article on predictive analytics tools and techniques cited above, vendors are finding ways to reduce the time and expertise involved in building predictive models. "What used to require weeks of writing code can now be accomplished with a few mouse clicks and a lot of automation on the back end," Lawton reported.
Advanced machine learning techniques are reducing the need to deeply understand how different variables affect each other, automatically choosing the best combination of algorithms for a given task. There is also a growing market of industry-specific analytics tools with prebuilt models and templates that embody best practices and dramatically simplify the predictive analytics process. Predictive analytics is becoming democratized, to use the industry parlance -- or that is the promise, at least.
Forrester Research principal analyst Boris Evelson, a leading expert on analytics who has covered this field for decades, has made these advanced analytics tools a chief area of his research focus. The embedded ML algorithms and conversational user interfaces, with natural language processing and natural language generation features, are potentially a game changer, he said. "So, not only do I no longer need a data scientist to make that calculation. I don't need a data scientist to explain it to me."
But it is early days, and these potentially wondrous tools are up against a persistent barrier. Two decades ago, Evelson said, new developments in BI software were heralded as "the greatest thing since sliced bread," offering point-and-click, drag-and-drop interfaces that put this then-advanced technology within reach of the user masses. That didn't happen.
"The number we like to use today is about 20% -- that is, no more than 20% of all enterprise decision-makers who could be using and should be using these tools are using them today," he said. "The rest are still relying on professional data analysts."
Technology is partly to blame, but it's mostly people, culture, process and data challenges that get in the way of wider adoption. With these new augmented analytics platforms, Evelson said, "is that 20% now going to be 30%, 40%, 50%? I don't know."
It's not just early, he added, but the benefits these automated intelligence platforms bring also carry risks. The machine learning algorithms and models are only as good as the data they're trained on. "If that data changes very rapidly, and it changes in a pattern that no longer looks like the data it was trained on -- well, professional data scientists understand this and work it into the process," Evelson said. But ModelOps "hasn't really made its way into these new augmented BI platforms yet." Updating these models can be a full-time job for a lot of people, he noted, suggesting that for now, at least, predictive analytics and other forms of advanced analytics is still a professional's game.
Jacqueline Biscobing, senior managing editor, News, and Ed Burns, former executive editor, contributed to this report.