What is data science? The ultimate guide
Data science is the field of applying advanced analytics techniques and scientific principles to extract valuable information from data for business decision-making, strategic planning and other uses. It's increasingly critical to businesses: The insights that data science generates help organizations increase operational efficiency, identify new business opportunities and improve marketing and sales programs, among other benefits. Ultimately, they can lead to competitive advantages over business rivals.
Data science incorporates various disciplines -- for example, data engineering, data preparation, data mining, predictive analytics, machine learning and data visualization, as well as statistics, mathematics and software programming. It's primarily done by skilled data scientists, although lower-level data analysts may also be involved. In addition, many organizations now rely partly on citizen data scientists, a group that can include business intelligence (BI) professionals, business analysts, data-savvy business users, data engineers and other workers who don't have a formal data science background.
This comprehensive guide to data science further explains what it is, why it's important to organizations, how it works, the business benefits it provides and the challenges it poses. You'll also find an overview of data science applications, tools and techniques, plus information on what data scientists do and the skills they need. Throughout the guide, there are hyperlinks to related TechTarget articles that delve more deeply into the topics covered here and offer insight and expert advice on data science initiatives.
Why is data science important?
Data science plays an important role in virtually all aspects of business operations and strategies. For example, it provides information about customers that helps companies create stronger marketing campaigns and targeted advertising to increase product sales. It aids in managing financial risks, detecting fraudulent transactions and preventing equipment breakdowns in manufacturing plants and other industrial settings. It helps block cyber attacks and other security threats in IT systems.
From an operational standpoint, data science initiatives can optimize management of supply chains, product inventories, distribution networks and customer service. On a more fundamental level, they point the way to increased efficiency and reduced costs. Data science also enables companies to create business plans and strategies that are based on informed analysis of customer behavior, market trends and competition. Without it, businesses may miss opportunities and make flawed decisions.
Data science is also vital in areas beyond regular business operations. In healthcare, its uses include diagnosis of medical conditions, image analysis, treatment planning and medical research. Academic institutions use data science to monitor student performance and improve their marketing to prospective students. Sports teams analyze player performance and plan game strategies via data science. Government agencies and public policy organizations are also big users.
Data science process and lifecycle
Data science projects involve a series of data collection and analysis steps. In an article that describes the data science process, Donald Farmer, principal of analytics consultancy TreeHive Strategy, outlined these six primary steps:
- Identify a business-related hypothesis to test.
- Gather data and prepare it for analysis.
- Experiment with different analytical models.
- Pick the best model and run it against the data.
- Present the results to business executives.
- Deploy the model for ongoing use with fresh data.
Farmer said the process does make data science a scientific endeavor. However, he wrote that in corporate enterprises, data science work "will always be most usefully focused on straightforward commercial realities" that can benefit the business. As a result, he added, data scientists should collaborate with business stakeholders on projects throughout the analytics lifecycle.
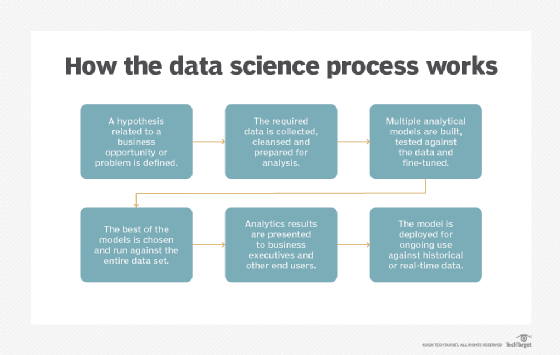
Benefits of data science
In an October 2020 webinar organized by Harvard University's Institute for Applied Computational Science, Jessica Stauth, managing director for data science in the Fidelity Labs unit at Fidelity Investments, said there's "a very clear relationship" between data science work and business results. She cited potential business benefits that include higher ROI, sales growth, more efficient operations, faster time to market and increased customer engagement and satisfaction.
Generally speaking, one of data science's biggest benefits is to empower and facilitate better decision-making. Organizations that invest in it can factor quantifiable, data-based evidence into their business decisions. Ideally, such data-driven decisions will lead to stronger business performance, cost savings and smoother business processes and workflows.
The specific business benefits of data science vary depending on the company and industry. In customer-facing organizations, for example, data science helps identify and refine target audiences. Marketing and sales departments can mine customer data to improve conversion rates and create personalized marketing campaigns and promotional offers that produce higher sales.
In other cases, the benefits include reduced fraud, more effective risk management, more profitable financial trading, increased manufacturing uptime, better supply chain performance, stronger cybersecurity protections and improved patient outcomes. Data science also enables real-time analysis of data as it's generated -- read about the benefits that real-time analytics provides, including faster decision-making and increased business agility, in another article by Farmer.
Data science applications and use cases
Common applications that data scientists engage in include predictive modeling, pattern recognition, anomaly detection, classification, categorization and sentiment analysis, as well as development of technologies such as recommendation engines, personalization systems and artificial intelligence (AI) tools like chatbots and autonomous vehicles and machines.
Those applications drive a wide variety of use cases in organizations, including the following:
- customer analytics
- fraud detection
- risk management
- stock trading
- targeted advertising
- website personalization
- customer service
- predictive maintenance
- logistics and supply chain management
- image recognition
- speech recognition
- natural language processing
- cybersecurity
- medical diagnosis
Learn more about eight top data science applications and associated use cases in an article by Ronald Schmelzer, principal analyst and managing partner at Cognilytica, a research and advisory firm that focuses on AI.
Challenges in data science
Data science is inherently challenging because of the advanced nature of the analytics it involves. The vast amounts of data typically being analyzed add to the complexity and increase the time it takes to complete projects. In addition, data scientists frequently work with pools of big data that may contain a variety of structured, unstructured and semistructured data, further complicating the analytics process.
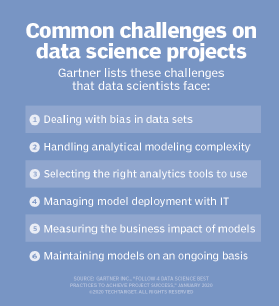
One of the biggest challenges is eliminating bias in data sets and analytics applications. That includes issues with the underlying data itself and ones that data scientists unconsciously build into algorithms and predictive models. Such biases can skew analytics results if they aren't identified and addressed, creating flawed findings that lead to misguided business decisions. Even worse, they can have a harmful impact on groups of people -- for example, in the case of racial bias in AI systems.
Finding the right data to analyze is another challenge. In a report published in January 2020, Gartner analyst Afraz Jaffri and four of his colleagues at the consulting firm also cited choosing the right tools, managing deployments of analytical models, quantifying business value and maintaining models as significant hurdles.
Read about four best practices for data science projects to help overcome the challenges in an article by Yujun Chen and Dawn Li, two data scientists at software development services firm Finastra.
What do data scientists do and what skills do they need?
The primary role of data scientists is analyzing data, often large amounts of it, in an effort to find useful information that can be shared with corporate executives, business managers and workers, as well as government officials, doctors, researchers and many others. Data scientists also create AI tools and technologies for deployment in various applications. In both cases, they gather data, develop analytical models and then train, test and run the models against the data.
As a result, data scientists must possess a combination of data preparation, data mining, predictive modeling, machine learning, statistical analysis and mathematics skills, as well as experience with algorithms and coding -- for example, programming skills in languages such as Python, R and SQL. Many are also tasked with creating data visualizations, dashboards and reports to illustrate analytics findings.
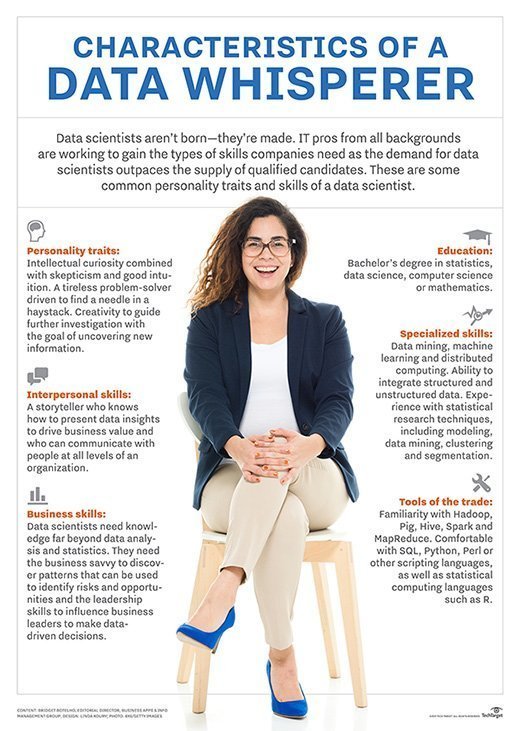
In addition to those technical skills, data scientists require a set of softer ones, including business knowledge, curiosity and critical thinking. Another important skill is the ability to present data insights and explain their significance in a way that's easy for business users to understand. That includes data storytelling capabilities for combining data visualizations and narrative text in a prepared presentation.
Get more details on must-have data science skills in an article by Kathleen Walch, another principal analyst and managing partner at Cognilytica.
Data science team
Many organizations have created a separate team, or multiple teams, to handle data science activities. As technology writer Mary K. Pratt explains in an article on how to set up a data science team, there's more to an effective team than data scientists themselves. It may also include the following positions:
- Data engineer. Responsibilities include setting up data pipelines and aiding in data preparation and model deployment, working closely with data scientists.
- Data analyst. This is a lower-level position for analytics professionals who don't have the experience level or advanced skills that data scientists do.
- Machine learning engineer. This programming-oriented job involves developing the machine learning models needed for data science applications.
- Data visualization developer. This person works with data scientists to create visualizations and dashboards used to present analytics results to business users.
- Data translator. Also called an analytics translator, it's an emerging role that serves as a liaison to business units and helps plan projects and communicate results.
- Data architect. A data architect designs and oversees the implementation of the underlying systems used to store and manage data for analytics uses.
The team commonly is run by a director of data science, data science manager or lead data scientist, who may report to either the chief data officer, chief analytics officer or vice president of analytics; chief data scientist is another management position that has emerged in some organizations. Some data science teams are centralized at the enterprise level, while others are decentralized in individual business units or have a hybrid structure that combines those two approaches.
Business intelligence vs. data science
Like data science, basic business intelligence and reporting aims to help guide operational decision-making and strategic planning. But BI primarily focuses on descriptive analytics: What happened or is happening now that an organization should respond to or address? BI analysts and self-service BI users mostly work with structured transaction data that's extracted from operational systems, cleansed and transformed to make it consistent, and loaded into a data warehouse or data mart for analysis. Monitoring business performance, processes and trends is a common BI use case.
Data science involves analytics applications that are more advanced. In addition to descriptive analytics, it encompasses predictive analytics that forecasts future behavior and events, as well as prescriptive analytics, which seeks to determine the best course of action to take on the issue being analyzed.
Unstructured or semistructured types of data -- for example, log files, sensor data and text -- are common in data science applications, along with structured data. Also, data scientists often want to access raw data before it has been cleaned up and consolidated so they can analyze the full data set or filter and prepare it for specific analytics uses. As a result, the raw data may be stored in a data lake based on Hadoop, a cloud object storage service, a NoSQL database or another big data platform.
Data science technologies, techniques and methods
Data science relies heavily on machine learning algorithms. Machine learning is a form of advanced analytics in which algorithms learn about data sets and then look for patterns, anomalies or insights in them. It uses a combination of supervised, unsupervised, semisupervised and reinforcement learning methods, with algorithms getting different levels of training and oversight from data scientists.
There's also deep learning, a more advanced offshoot of machine learning that primarily uses artificial neural networks to analyze large sets of unlabeled data. In another article, Cognilytica's Schmelzer explains the relationship between data science, machine learning and AI, detailing their different characteristics and how they can be combined in analytics applications.
Predictive models are another core data science technology. Data scientists create them by running machine learning, data mining or statistical algorithms against data sets to predict business scenarios and likely outcomes or behavior. In predictive modeling and other advanced analytics applications, data sampling is often done to analyze a representative subset of data, a data mining technique that's designed to make the analytics process more manageable and less time-consuming.
Common statistical and analytical techniques that are used in data science projects include the following:
- classification, which separates the elements in a data set into different categories;
- regression, which plots the optimal values of related data variables in a line or plane; and
- clustering, which groups together data points with an affinity or shared attributes.
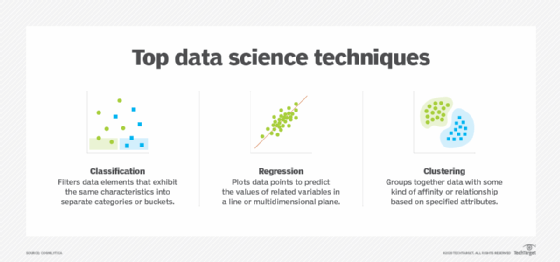
Data science tools and platforms
Numerous tools are available for data scientists to use in the analytics process, including both commercial and open source options:
- data platforms and analytics engines, such as Spark, Hadoop and NoSQL databases;
- programming languages, such as Python, R, Julia, Scala and SQL;
- statistical analysis tools like SAS and IBM SPSS;
- machine learning platforms and libraries, including TensorFlow, Weka, Scikit-learn, Keras and PyTorch;
- Jupyter Notebook, a web application for sharing documents with code, equations and other information; and
- data visualization tools and libraries, such as Tableau, D3.js and Matplotlib.
In addition, software vendors offer a diverse set of data science platforms with different features and functionality. That includes analytics platforms for skilled data scientists, automated machine learning platforms that can also be used by citizen data scientists, and workflow and collaboration hubs for data science teams. The list of vendors includes Alteryx, AWS, Databricks, Dataiku, DataRobot, Domino Data Lab, Google, H2O.ai, IBM, Knime, MathWorks, Microsoft, RapidMiner, SAS Institute, Tibco Software and others.
Get more information on top data science tools and platforms in an article by tech writer Pratt.
Careers in data science
As the amount of data generated and collected by businesses increases, so does their need for data scientists. That has sparked high demand for workers with data science experience or training, making it hard for some companies to fill available jobs.
In a survey conducted in 2020 by Google's Kaggle subsidiary, which runs an online community for data scientists, 51% of the 2,675 respondents employed as data scientists said they had a master's degree of some kind, while 24% had a bachelor's degree and 17% had a doctorate. Many universities now offer undergraduate and graduate programs in data science, which can be a direct pathway to jobs.
An alternative career path is for people working in other roles to be retrained as data scientists -- a popular option for organizations that have trouble finding experienced ones. In addition to academic programs, prospective data scientists can take part in data science bootcamps and online courses on educational websites like Coursera and Udemy. Various vendors and industry groups also offer data science courses and certifications, and online data science quizzes can test and provide basic knowledge.
As of December 2020, the Glassdoor job search and company reviews site listed an average base salary of $113,000 for data scientists in the U.S., with a range of $83,000 to $154,000; the average salary for a senior data scientist was $134,000. On the Indeed jobs site, the average salaries were $123,000 for a data scientist and $153,000 for a senior data scientist.
How industries rely on data science
Before they became technology vendors themselves, Google and Amazon were early users of data science and big data analytics for internal applications, along with other internet and e-commerce companies like Facebook, Yahoo and eBay. Now, data science is widespread in organizations of all kinds. Here are some examples of how it's used in different industries:
- Entertainment. Data science enables streaming services to track and analyze what users watch, which helps determine the new TV shows and films they produce. Data-driven algorithms are also used to create personalized recommendations based on a user's viewing history.
- Financial services. Banks and credit card companies mine and analyze data to detect fraudulent transactions, manage financial risks on loans and credit lines, and evaluate customer portfolios to identify upselling opportunities.
- Healthcare. Hospitals and other healthcare providers use machine learning models and additional data science components to automate X-ray analysis and aid doctors in diagnosing illnesses and planning treatments based on previous patient outcomes.
- Manufacturing. Data science uses at manufacturers include optimization of supply chain management and distribution, plus predictive maintenance to detect potential equipment failures in plants before they occur.
- Retail. Retailers analyze customer behavior and buying patterns to drive personalized product recommendations and targeted advertising, marketing and promotions. Data science also helps them manage product inventories and their supply chains to keep items in stock.
- Transportation. Delivery companies, freight carriers and logistics services providers use data science to optimize delivery routes and schedules, as well as the best modes of transport for shipments.
- Travel. Data science aids airlines with flight planning to optimize routes, crew scheduling and passenger loads. Algorithms also drive variable pricing for flights and hotel rooms.
Other data science uses, in areas such as cybersecurity, customer service and business process management, are common across different industries. An example of the latter is assisting in employee recruitment and talent acquisition: Analytics can identify common characteristics of top performers, measure how effective job postings are and provide other information to help in the hiring process.
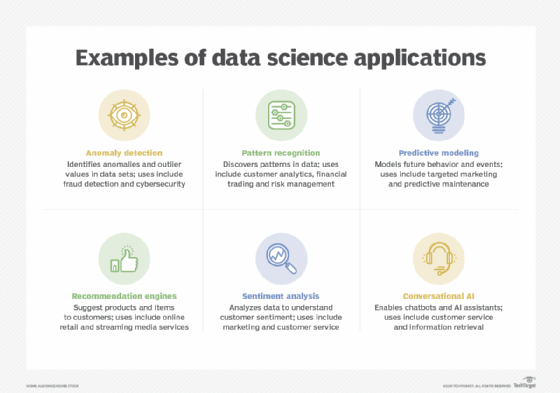
History of data science
In a paper published in 1962, American statistician John W. Tukey wrote that data analysis "is intrinsically an empirical science." Four years later, Peter Naur, a Danish software programming pioneer, proposed datalogy -- "the science of data and data processes" -- as an alternative to computer science. He later used the term data science in his 1974 book, Concise Survey of Computer Methods, describing it as "the science of dealing with data" -- though again in the context of computer science, not analytics.
In 1996, the International Federation of Classification Societies included data science in the name of the conference it held that year. In a presentation at the event, Japanese statistician Chikio Hayashi said data science includes three phases: "design for data, collection of data and analysis on data." A year later, C. F. Jeff Wu, a university professor in the U.S. who was born in Taiwan, proposed that statistics be renamed data science and that statisticians be called data scientists.
Read about 13 books on data science that will boost your knowledge of issues, tools and techniques.
American computer scientist William S. Cleveland outlined data science as a full analytics discipline in an article titled "Data Science: An Action Plan for Expanding the Technical Areas of Statistics," which was published in 2001 in the International Statistical Review. Two research journals focused on data science were launched in the next two years.
The first use of data scientist as a professional job title is credited to DJ Patil and Jeff Hammerbacher, who jointly decided to adopt it in 2008 while working at LinkedIn and Facebook, respectively. By 2012, a Harvard Business Review article co-written by Patil and American academic Thomas Davenport called data scientist "the sexiest job of the 21st century." Since then, data science has continued to grow in prominence, fueled partly by increased use of AI and machine learning in organizations.
Future of data science
As data science becomes even more prevalent in organizations, citizen data scientists are expected to take on a bigger role in the analytics process. In its 2020 Magic Quadrant report on data science and machine learning platforms, Gartner said the need to support a broad set of data science users is "increasingly the norm." One likely result is increased use of automated machine learning, including by skilled data scientists looking to streamline and accelerate their work.
Dig further into the field by following these data science blogs.
Gartner also cited the emergence of machine learning operations (MLOps), a concept that adapts DevOps practices from software development in an effort to better manage development, deployment and maintenance of machine learning models. MLOps methods and tools aim to create standardized workflows so models can be scheduled, built and put into production more efficiently.
Other trends that will affect the work of data scientists going forward include the increasing push for explainable AI, which provides information to help people understand how AI and machine learning models work and how much to trust their findings in making decisions, and a related focus on responsible AI principles designed to ensure that AI technologies are fair, unbiased and transparent.